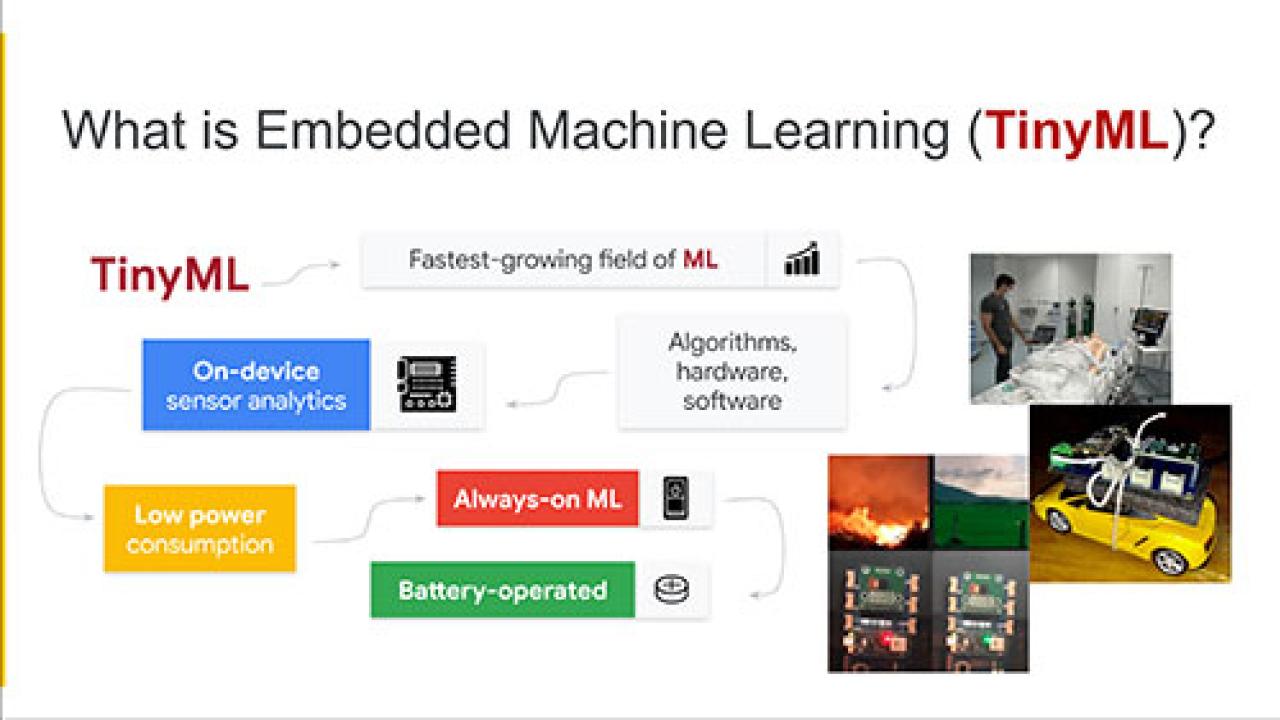
Running machine learning on cheap, low consumption electronic devices could really empower the way science is done, especially in developing countries. This so-called "Tiny Machine Learning", which requires low power and can be harnessed at a low cost, was the topic of a recent workshop organised by ICTP's Science, Technology and Innovation(STI) Unit that attracted hundreds of participants from throughout the developing world.
The purpose of the workshop, titled "SciTinyML: Scientific Use of Machine Learning on Low-Power Devices", was to disseminate the know-how and create a network which facilitates the exchange of knowledge and data. “We brought together 20 universities from around the globe that either already work in this field or are interested in working in this field," says Marco Zennaro, STI's coordinator. The workshop, preceded by some preliminary seminars, was held from 18 to 22 October, and hosted 200 participants, ten from each university.
To understand what TinyML is, it is useful to get an idea of how machine learning works. Have you ever wondered how your smartphone, or your computer can automatically detect spam e-mails, know your musical tastes or your shopping habits? And what about voice recognition or self-driving cars? The answer is machine learning (ML), namely the branch of artificial intelligence that designs software capable to recognise patterns within a large set of data. For example, imagine trying to configure a device to be able to distinguish between pictures of cats and dogs. The first step would be to furnish the software with a large number of pictures of both, labelled as cats or dogs. The software in this phase, called training, learns how to tell one from the other. In a second phase one can insert new unlabelled data and the software is now able to tell if it is processing a picture of a cat or a dog.
The applications of this technology are not limited to what your devices know about you. They are extremely useful in scientific fields in which often a human action is not capable alone to extract sense from large amounts of data.
In standard machine learning, the computational power needed to generate the output is very high. Typically, the collected data is sent via the Internet to huge data centres where it is processed then the output is sent back to the device. With TinyML this process is no longer needed, and the information can be processed on-site.
“The basic idea behind TinyML is to have machine learning models that, instead of running on very powerful computers, run on very small and cheap microcontrollers,” explains Zennaro. “This has a lot of advantages in general,” he continues. “Half of the world is still not connected to the Internet, so sending the data around is unthinkable. These devices are low cost and very low power: with a small battery they can go on for months, if not years. So, it also solves the issues of the lack of energy in many of these countries.”
One other advantage is that the data are elaborated where they are measured. This allows scientists to build datasets that are meaningful in the region of the world where the research is carried out: “The applications are more interesting and useful for developing countries. Since you collect data and take measurements, you can take them about phenomena which happen in the countries themselves. There is an example of a student from the Institut de Mathématiques et de Sciences Physiques, in Benin, who applied TinyML to understand plant diseases in his home country. Taking a picture of the plant, you can understand if a disease is present. If he had worked with standard ML, the open datasets available on which to create his models are relative to plants form the northern hemisphere, while he is interested in cotton or other plants that are present in the south.” Furthermore, Zennaro adds: “From the educational point of view, working with these devices allows the students to apply ML on a device that they have in their hand and not on a server which is probably located in the USA or Europe. So they feel that this device belongs to them, a technology of which they are the owners.”
Among the workshop participants was Professor Jimmy Nsenga, a software engineer and visiting senior lecturer at the African Excellence Centre in Internet of Things in Kigali, Rwanda. Professor Nsenga already has experience with TinyML, and is using his knowledge to train students in Africa. He gives a good example of TinyML in action, through the thesis work of one of his master's students.
The thesis project provided for the use of TinyML for the detection in the water of bacteria that cause cholera. “At the continental level, Africa has the highest global cholera burden, counting up to 54% of 1.3 to 4.0 million cases of cholera per year, and 21,000 to 143,000 deaths worldwide,” explains Nsenga, “Back in 2017, the Guardian had reported that in 41 countries in the world (most of them in Africa), a fifth of people drink water from a source that is not protected from contamination. In the same report, it is also noted that even for water sources which were identified to be of ‘improved safety’, 91% of them were still containing escherichia coli bacterial pathogens. This raises the need to monitor the water quality at the end-user tap.”
Nsenga explains how, even though most of the countries have invested in well performing water treatment centres, the quality of the water can be degraded while transiting from treatment centres to the end-user taps: “What counts is the quality at the point of collect,” he says. To envision water mass deployment from the centre to the rural areas requires a very expensive infrastructure. “Our solution overcomes this challenge by developing an affordable, small and lightweight Internet of Things device that can be plugged into the tap in a non-invasive way and therefore monitor the quality of the tap water in real-time without accessing the Internet. It is important to note that the test results should quickly warn the end-consumer about the water quality.”
Professor Nsenga underlines the decisive action of TinyML in the development of this technology. A solution designed with standard techniques would need a continuous wireless communication, and this, he explains, has three main challenges in the rural context: it is too expensive, it consumes too much energy, and it has decision latency, while in these cases the user needs to be warned as soon as possible.
“It is for solving all those challenges that TinyML comes to the rescue, as it deploys optimised light ML models on energy- and processing -constrained devices, therefore enabling to run cholera detection analytics offline and rapidly,” says Nsenga.
Professor Marcelo Rovai, one of the Workshop lecturers, started teaching courses on TinyML at the Federal University of Itajubá (UNIFEI), Brazil. Since the beginning of 2021, he has been working closely with Zennaro as an active member of the TinyML4D group (link: http://tinymledu.org).
The students who attended his courses in Brazil have developed many interesting projects using TinyML, and even though these works have a didactic purpose, they shed light on the potential of the technology. The projects range from the recognition by images of forest fires, to models for COVID-19 detection trained with captured audio of coughs. This last project was motivated by the fact that “some communities either still do not have full access to COVID-19 tests or need more tests to combat the pandemic,” Rovai explains.
“Some students decided to go on and develop more serious and real projects that should generate papers to be defended in their final engineering courses to be held next December,” he says.
Among these is a project to develop small and economic ECG devices to detect cardiac arrhythmias and another regarding the recognition of diseases of coffee plants by image analysis.
Rovai comments: “ICTP has an important role in bringing these technologies to developing countries. Students and academics from more than 40 universities are participating in the seminars and workshops that have been organized by ICTP," he says, adding, "This kind of initiative is bringing excitement and helping countries to have access to new technologies that can solve their problems, without the use of complex and very expensive infrastructures.”
So far, the use of TinyML still has some significant limitations: “From an outreach perspective, we need to educate the broader community about this technology,” claims Professor Vijay Janapa Reddi, associate professor in the John A. Paulson School of Engineering and Applied Sciences (SEAS) at Harvard University and lecturer at the workshop. “Without proper education, it is hard to scale. Building some on-device ML and showing it to the world as a demo is one thing. That's useful for inspiring students. But if we want to build the next generation of engineers, we need to think about the pedagogical underpinnings of enabling ML at scale. This means we need a curriculum in schools that fits what industry wants with the rigor that academics traditionally provide,” he says.
There are also technical issues. Zennaro explains: “It is true that these devices consume little energy, but only when they work to understand what is happening in the field. The training part still requires big computers, and so, a lot of energy.” He adds, “These microcontrollers have very little memory and very low computational power. They are equivalent to computers from 25 to 30 years ago. So, they are limited. There is a lot of research to understand how the miniaturisation of the model does not affect the results.” Another aspect to consider is that the hardware systems used to run TinyML algorithms are embedded, which means that they are designed for a specific task, and they are not reprogrammable. For this reason, Zennaro says, “For the workshop, we furnished tested hardware devices, not because they are expensive, but because it is important that everybody possesses the same devices to facilitate the exchange of data and machine learning models. This promotes cooperation.”
Promoting cooperation is, in fact, a crucial point for this initiative: “We are forming a community on Discord, a social network usually used by gamers, which is now becoming more and more used in the academic environment. In this TinyML network there are about 300 people who exchange papers and various information.” says Zennaro.
Professor Nsenga underscores the importance of creating a community: “The networking of participants enables them to share knowledge and experience,” he says, adding, “This collaboration may facilitate the collection and sharing of more data from a diversity of contexts, enabling us to train more robust TinyML analytics.” He continues: “Africa still not only lacks enough data scientists and enough embedded engineers (engineers trained to design and develop embedded systems and the dedicated software), but also, unfortunately, the knowledge and education gap is even wider when it comes to TinyML, which requires both embedded engineering and ML skills. This ICTP initiative will play an important role to reduce this gap. Providing free TinyML-enabled development boards to participating universities is a simple but valuable contribution to enable equal opportunities to learn TinyML, not only on virtual simulators but also on real devices.”
--Luca Papapietro